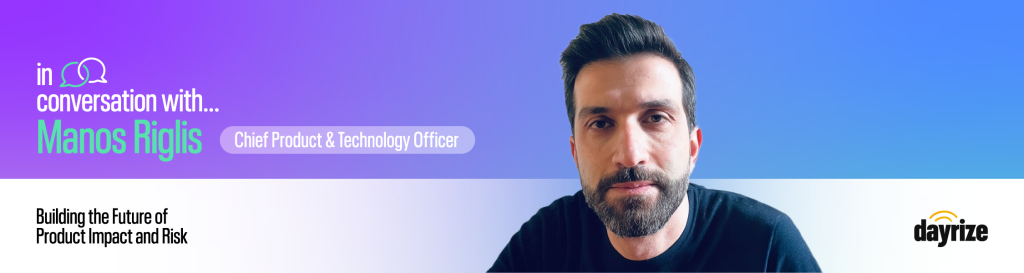
Manos Riglis, our Chief Product & Technology Officer, combines deep, hands-on engineering skill with big-picture vision to turn bold ideas into measurable, data-driven results. In this interview, he traces his journey from agency coder to mission-led product strategist, explains how AI is transforming analytics, and reveals what’s next for supply-chain transparency, impact measurement, and Dayrize’s evolving data platform. Let’s dive in!
What first ignited your passion for strategy and technology, and how did that path ultimately lead you to your role today as Chief Product & Technology Officer at Dayrize?
I got into technology because I enjoy building things – finding solutions to problems and creating tools people find useful. I started my career in digital agencies, building applications for a range of clients and eventually leading a team of 40. Over time, I became more interested in shaping products, not just executing someone else’s vision.
That led me to a company building data and analytics platforms for natural resource commodities, where I got more involved in both technology and organizational strategy, especially as we scaled the team from 30 to 120 engineers. I enjoyed working across all levels, from architecture to onboarding and innovation.
After that, I joined Trustpilot as VP of Engineering, then Product, focusing on fraud analytics. I stayed through their Series E to IPO. I then moved to Amazon, working in their advertising business on targeting solutions using data and machine learning.
Eventually, I tried launching my own adtech startup. While we didn’t find product-market fit, it helped clarify what I wanted next: a startup with a real mission and room for innovation. When I met the team at Dayrize, I knew it was the right fit, working at the intersection of impact, AI, and data, where I felt I could make a meaningful contribution.
How do you see artificial intelligence reshaping the data-analytics landscape, and what new opportunities and hurdles do you think will arise along the way?
This is what we’re trying to figure out now. AI, and especially generative AI, feels like a natural fit for analytics. It adds the capability to reason and then take action. That ability to formulate a plan and act on it is what really stands out.
It has the potential to streamline analytics going forward. A lot of times in analytics, you’re trying to determine, “Where are we today?” – that’s descriptive analytics. For example, “This is where a product’s impact measurement stands.”
But what’s really interesting is how AI can help make that information actionable. Can we say, “Based on this data, what steps should we take next?” There are now a lot of techniques, through generative AI and traditional machine learning, that allow us to build forecast models or simulations.
AI brings those techniques into the market, making them more accessible. Another area that’s exciting is agentic architectures, basically agents that can act on your behalf. In our case, we’re exploring whether agents can understand regulations and then run the right analytics to generate insights for customers.
There’s a lot of opportunity here, and the pace of innovation is breakneck. Every day there’s a new tool, a new technique.
But with all that, there are risks, things like explainability, observability, and putting guardrails in place. So even as we open up new use cases, we need to make sure the answers are correct, unbiased, and trustworthy. It’s a different way of building software, and although there’s a lot of potential, we have to manage those risks carefully.
Since our last interview, DaySI has gained momentum. Can you share the latest developments in the project and any key milestones we’ve achieved?
Right now, we’ve iterated on our data pipeline. We’re using inference to fill in data gaps, when we have missing data, we can infer it. We’ve also run accuracy tests to understand where we are, and what the expected level of accuracy is based on benchmark data.
We’ve made updates based on customer feedback, which is super useful. When customers point out where something isn’t working well, that helps us prioritize our next steps.
Beyond that, we’ve started work on agents, our first one is focused on reasoning over product data to generate insights or suggest improvements. In parallel, we’ve kicked off a project to build out our data platform and begin ingesting a large number of external datasets. That will give our agents the context they need to provide more valuable analytics.
So right now, we’re focused on two areas: strengthening the data side, and building out the agent flows. Going forward, we’re focusing more on the supply chain. That’s valuable for two reasons—first, because a lot of product impact originates from manufacturing, and second, because understanding your supply chain is crucial for risk analytics. It helps us understand where materials are coming from and what the exposure is.
What trends and innovations do you believe will define the future of sustainability analytics in business?
One major trend is better-quality data. When it comes to supply chains, data is often locked away in silos, which hurts transparency.
So a key area of innovation will be: how can we use publicly available data and AI to generate insights or point out hotspots that customers should focus on?
Another important area is simulation. If you can simulate changes to your supply chain—like modifying a manufacturing process or switching factories – you can make more informed decisions. Instead of relying on historical data alone, you can get more accurate, forward-looking metrics.
And it’s not just about CO2 emissions. Let’s say you move production to a location with lower emissions, but the materials come from a country with high deforestation rates. There are trade-offs. So being able to model these impacts holistically will be important.
It all comes back to improving transparency and using data to generate real, actionable insights.
What advice would you give to companies struggling to scale their impact measurement efforts?
What I see often is that the data needed for impact measurements is diverse and scattered across silos. In many cases, it requires collaboration, with suppliers and partners, to get a complete picture.
It can be a painful process, but investing in transparency leads to better decision-making. Companies should not only invest in collecting data but also work to close the gaps.
Something is better than nothing, it gets you started on the journey. Once you’re moving, it becomes easier to improve and refine.
To keep up to date with our latest releases and get to know the team behind Dayrize, subscribe to our updates below and follow us on LinkedIn.